Introduction
In today’s fast-paced business environment, companies increasingly rely on data-driven solutions to enhance financial efficiency and streamline operations. Accounts Receivable (AR) is a critical function, and optimizing its management can greatly enhance a company’s cash flow and revenue predictability. By leveraging Machine Learning (ML) technologies, organizations can gain deeper insights into customer payment patterns, improve collection processes, and forecast future sales more accurately. At the DATA Center of Excellence, we’ve implemented ML-driven solutions for AR data, transforming the way our clients manage their finances.
Problem Statement
Organizations often face significant challenges in managing AR data. Manual processes and static reporting make it difficult to predict customer payment behaviors, leading to delayed payments and inaccurate financial forecasts. The lack of real-time insights into customer segments, on-time payments, and sales trends can result in missed opportunities, cash flow problems, and inefficient collection strategies.
To address these challenges, companies need a solution that provides accurate payment predictions, identifies high-risk customers, and improves overall financial planning. This is where machine learning comes into play.
Why do we need to implement Machine Learning?
Machine Learning offers several benefits that traditional methods of AR management cannot match. Some of the key advantages include:
- Predictive Accuracy: ML models such as Logistic Regression and XGBoost improve the accuracy of payment predictions and forecasting, enabling proactive decisions.
- Customer Segmentation: Using K-means clustering, businesses can segment customers based on their payment behaviors, targeting high-risk customers and optimizing collection strategies.
- Time Utilization: By automating forecasting and payment prediction processes, organizations can save time and allocate resources more efficiently.
- Proactive Financial Management: ML implementations enable businesses to foresee late payments, identify patterns, and mitigate risks before they impact cash flow.
Architecture
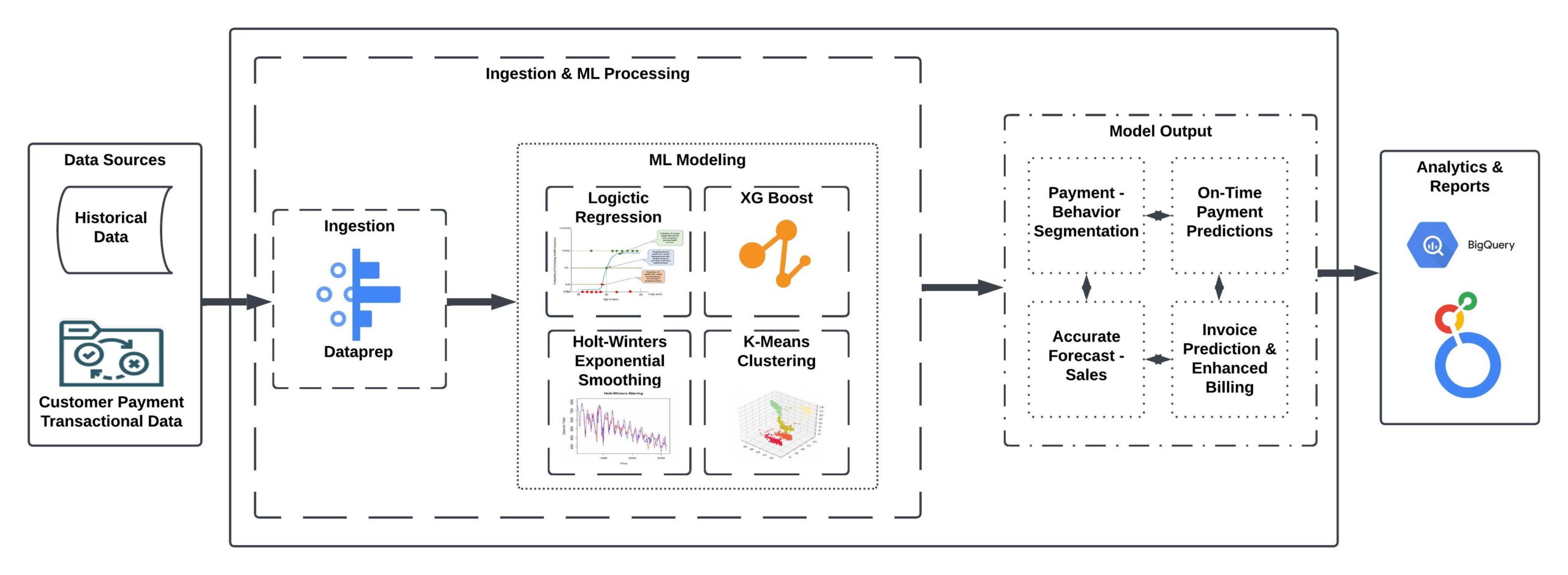
Our Approach and Client Value Add
At the DATA Centre of Excellence, our approach focuses on tailoring machine learning models to the specific financial needs of our clients, particularly within the AR domain. By leveraging advanced ML techniques, we were able to address key customer challenges and deliver significant value.
-
Deep Feature Engineering
We thoroughly analyzed our client’s dataset to engineer features that would best represent AR patterns. For example, we created features such as days_to_payment, outstanding_balance, and on_time_payment to capture the intricacies of payment behavior. We also normalized features for consistency, ensuring that models like K-Means and XGBoost could process the data effectively.
-
Comprehensive Payment Behavior Analysis
We designed multi-model classification to categorize customers into risk-based segments—high-risk, medium-risk, and low-risk—based on payment delays and outstanding amounts. This helped the client focus their collection efforts more efficiently, leading to faster payment recovery and a more structured AR management process.
-
Actionable Sales Forecasting
Our Holt-Winters Exponential Smoothing model provided highly accurate sales forecasts, projecting peak sales of $85.80 million in March 2025. These forecasts enabled our client to optimize their inventory levels, reduce overhead costs, and align their procurement strategy with expected demand.
-
Improved On-Time Payment Predictions
By implementing Logistic Regression, we provided our client with accurate predictions of whether a customer would pay on time, helping them proactively manage cash flow. For example, using payment history, division, and invoice amounts, we achieved 90% accuracy in predicting on-time payments.
-
Increased Billing Precision
Using XGBoost, we accurately predicted invoice amounts based on factors like order_amount, tax, and division. This helped improve billing accuracy, leading to fewer disputes, faster invoice processing, and higher client satisfaction.
Customer-Centric Value
By applying K-Means Clustering for customer segmentation, the client could target high-value customers for improved relationship management and offer customized payment plans to medium- and low-value customers. This not only helped in maintaining strong client relationships but also led to increased retention and higher recovery rates.
The impact was an immediate reduction in overdue payments by 15%, faster collections by 20%, and improved cash flow management. Our client was able to allocate their resources more effectively, enhancing both their operational efficiency and financial performance.
Conclusion
Machine Learning is transforming financial management by delivering predictive capabilities and actionable insights that go beyond the reach of traditional methods. When applied to Accounts Receivable (AR) data, ML models can significantly improve cash flow optimization, enhance revenue forecasting accuracy, and streamline collection processes. At the DATA Center of Excellence, our ML-driven solutions have empowered clients to revolutionize their AR management, resulting in better financial performance and increased operational efficiency. As organizations continue to adopt data-driven strategies, integrating machine learning will be essential for maintaining a competitive edge and achieving sustainable financial success.
If you’re grappling with delayed payments, inefficient collections, or uncertain forecasting, our team of technical experts at the DATA Center of Excellence is ready to collaborate with you and explore how our machine-learning-driven solutions can help streamline your AR management and enhance financial performance.